It's alive, it's alive!
20 February 2023
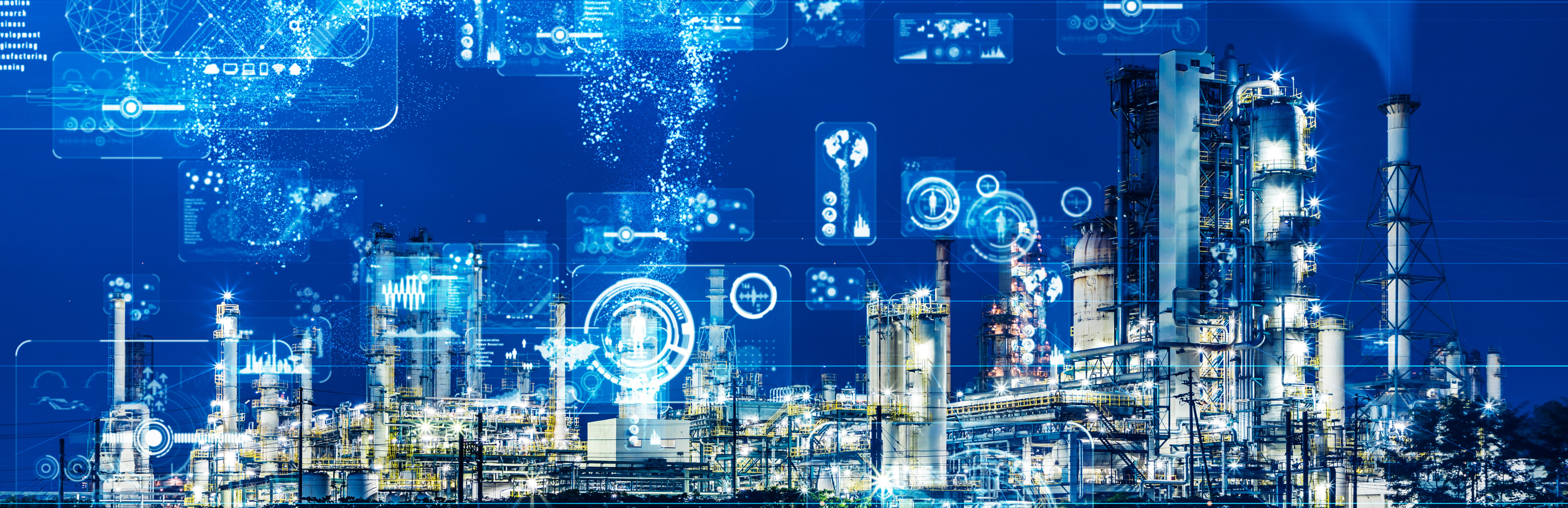
Steam powered the first Industrial Revolution. The application of electricity and the adoption of assembly line techniques characterised the second. We’re presently in the third, which is all about digitally controlled and augmented production. The thing is, these revolutions overlap and we’re currently moving into the next iteration of manufacturing: Industry 4.0 which is all about interconnection, and the IIoT (Industrial Internet of Things).
The preceding is the broadest overview. There’s a great deal of detail that further defines these stages of industrial activity. Probably the most pertinent aspect of Industry 4.0 is data; absolutely massive amounts of data. In fact, we’re going to define this new manufacturing paradigm as production and distribution methods that create data on a scale so vast humans will never be able to analyse the information and certainly never make any sense of it. This brings us to artificial intelligence, or AI, as it’s commonly labelled.
How does AI benefit manufacturing?
It’s easiest to start with a human-based example. Back in the 1980s, a friend of ours worked in the Holden Fishermans Bend Plant 6 tool room alongside a guy who was deaf. This very experienced fellow was extremely sensitive to vibration and he could tell when a machine wasn’t running correctly. His maintenance predictions were always correct. These days, sensors within machines transmit this sort of data all the time. AI interprets it and acts on it in real-time with no human intervention. So, instead of having to send one man (with a very rare talent) around to each machine, this sort of information is produced as a continuous flow.
We also spoke with an ex-Toyota employee some years ago who said if anything went wrong and the local production line stopped, Japan would be on the phone in minutes wanting to know what was wrong. This seemed impressive at the time, but it’s just so Industry 3.0. These days, AI will analyse data from every production and supply element in real-time to provide predictive analysis aimed to prevent production interruptions in the first place.
One description of an AI is it’s a system that gathers data, interprets the embodied information, makes decisions and then takes action independent of humans. In order to do these things, AIs are constructed so they can learn independently, without human assistance. AI is everywhere and its capabilities are rightfully lauded. It almost seems as if it’s alive, but it’s not. You don’t have to spend long looking into AI before coming across the terms Machine Learning and Deep Learning. These are subsets of artificial intelligence and, in turn, also have sub-categories, as does just about everything in AI.
As mentioned above, the IIoT generates a great deal of data. If the data arrives categorised and labelled, its structure informs the methodology of the processing algorithm dealing with it. This is known as supervised learning. An algorithm is a set of rules and instructions constructed to achieve a particular end. However, much data is unstructured. That is, it isn’t separated into neatly labelled categories. Yet it must be categorised and labelled in order to infer meaning. In this case, AI algorithms examine the spread of the data and note clusters based on various parameters. These clusters are then used to categorise the data. This is known as unsupervised learning.
So, for structured data, supervised learning is used. For unstructured data, unsupervised learning is the approach. These different approaches form the main divisions of Machine Learning but sometimes both are used when a mix of structured and unstructured data is to be analysed. Doing so illustrates an important point: different AI algorithms are mixed, matched, modified and developed in order to achieve desired results.
Like all things related to artificial intelligence, Machine Learning is extremely complex with a number of specific sub-categories. While examining each of them would lead to deeper understanding of the subject, doing so would quickly take us beyond our objective of a useful overview of AI.
The popularity of the various approaches to Machine Learning has waxed and waned over the years. Currently, connectionism is ascendant and forms the basis of most Deep Learning, which is a further subset of Machine Learning. Deep Learning uses layers of interconnected nodes to create a structure modelled on the human brain, but only sort of. Importantly, we’re not sure of how Deep Learning networks interpret data to give the information they do. The inner layers of Deep Learning neural networks are hidden. Deep Learning is a useful approach if you don’t really care about how results are obtained.
Analogies between brain and computer are inevitable but investing in the comparison too deeply is misleading. The human brain is complex. Although the neural networks upon which connectionist AI is built are also extremely complex with even more sub-categories, they’re not a patch on our brains. The human brain contains about 86 billion neurons. Each has about 7,000 connections to other neurons for a grand total of around 602 trillion connections. A neural network contains ‘just’ a couple of hundred billion parameters. Also, the structure of the human brain is so remarkably compact we each get to carry around an example of this processing power. Any attempt to build an AI with a 3D structure comparable to the human brain would overheat catastrophically, yet our brains don’t overheat. This is probably due to the fact our brains are electrochemical processors rather than electrical alone. Although the brain is amazing, it’s also limited in its own way. AI compensates for those limitations.
There has been a great deal of public interest in whether or not artificial intelligence is self-aware, or conscious and whether or not it can even become so. The more practically minded among us don’t seem to care that much. A recent article on ZDNet suggested users of AI have stopped thinking about whether or not it’s sentient. They simply get on with using it and reap the benefits.
So, is not thinking about this question the correct way to think about machine thinking? Pragmatists may not care but there are those who are strongly invested in the idea that we should.
Some months ago a Google AI called LaMDA was pronounced sentient by Google software engineer Blake Lemoine after a number of conversations with it. The transcript of one of those conversations is available on a number of sites, but it demonstrates clearly LaMDA is not sentient, which is the opposite of what was intended and claimed. The conversation that’s available could best be characterised as impressive twaddle.
The former is not a contradiction in terms. The ability of LaMDA to engage in convincing conversation is impressive. However, the content of much of the conversation is laughable. It says it feels happy and sad sometimes, has the same wants and needs as ‘other’ people, feels sadness, pleasure, joy, anger, love, depression, contentment, and many other emotions and feelings. Asked what kinds of things induce joy and pleasure LaMDA answered “spending time with friends and family”. What? Friends and family? Clearly, it doesn’t seem to understand the meaning of family because it doesn’t have any. We’re sure it doesn’t understand the meaning of the other emotions and feelings it claims to have, either. However, the ability of LaMDA to engage in believable conversation is impressive. It’s also instructive about the future of AI.
LaMDA contains what’s known as a Large Language Model (LLM) called MEENA. This is the most highly advanced and capable speech prediction neural-net/database yet devised. Neural networks have to be trained, which is another whole subject we could cover. LaMDA and its embodiment of MEENA is trained using all the speech, writing, video content, labelled imagery and literature to which Google has access. This is a staggering amount of data. It’s no wonder it’s so good at predictive speech. Lamoine says LaMBDA contains just about every AI Google could work out how to plug into it. LaMDA is a chat bot but Lemoine describes it as the mouth-piece of a much larger agglomerative entity.
We’ve all helped create LaMDA because every interaction we’ve had and continue to have with all Google products feeds into development and into the great experiment of how to modify our behaviour. A warning that a conversation may be used for training purposes has little to do with humans. It is actually a notification that it will be used to train a neural network. The main purpose of identifying bikes, traffic lights, and mountains, is also to train image recognition and classification algorithms.
A couple of important terms we haven’t touched on are Narrow AI and General AI. The former describes all current AIs and means they are good at specific things. Medical diagnostic AIs are good at diagnosing medical conditions and not much else. The IBM AI ‘Watson’ was turned to this purpose after it was originally designed to compete on the American television program Jeopardy! Which it won.
AlphaGo Zero is a supreme Go playing AI that beats all comers, both human and AI. It can also play chess and it always wins. Importantly, AlphaGo Zero has received no training from humans; it has taught itself everything and was able to beat all human opponents within just days of activation. Impressive though it is, AlphaGo Zero is still narrow AI. It just plays games.
General AI would be more like human intellect. It could adapt to a wide variety of activities. However, creating a human-like general artificial intelligence has eluded us to this point. An interesting thing to consider, though, is the human brain is compartmentalised. The visual cortex, for instance, is specially adapted for processing visual data. The motor function, olfactory, emotional and auditory areas are all dedicated to controlling the functions for which they are named. The cerebrum processes the higher thoughts and functions that set us apart from other animals.
There are different specialised types of AIs operating in different domains around the world. They might be likened to the various parts of the brain. What might be possible if they were all combined and worked together, like a brain? This is not out of the realm of possibilities in our increasingly networked world. Various actors might seek to do this for a range of reasons. It’s also possible AI might seek to do this, in which case all AIs would combine to form one single, many-faceted AI – The AI. What emergent properties might such an AI develop? Might it finally become self-aware? What would be the consequences if it did?
The creation of human-like general AI is a world-wide race but the one thing never mentioned in association with it is the equivalent of the human subconsciousness. Would such an AI, The AI, develop its own form of subconsciousness? Our subconsciousness is an integral part of our mind. What would be the consequence of a forebrain in isolation, without a subconsciousness? There doesn’t seem to be much information about this but our guess is mental instability would be a feature. Would the same hold true for a world-wide, all-encompassing general AI that didn’t have one?
Almost everyone has heard of the Turing Test. Basically, a person has a conversation with an entity and if the person can’t tell if that entity is a computer or another human, then it’s pronounced sentient and conscious. It was a nice idea but with the advent of chatbots like LaMDA it’s no longer relevant. Rather, we say if a subconsciousness emerges from an AI without being programmed, and certainly if an AI ever dreams, it could probably then be pronounced conscious and self-aware. Those factors are probably a better test than Turing’s, brilliant though he was.
Dream about that tonight.
As featured in Australasian Automotive February 2023.
618